MS05 - OTHE-2 Interfaith Prayer and Reflection Room (#3020C) in The Ohio Union
Preparing for the Next Pandemic: Modeling and Simulation in Drug Development
Wednesday, July 19 at 10:30am
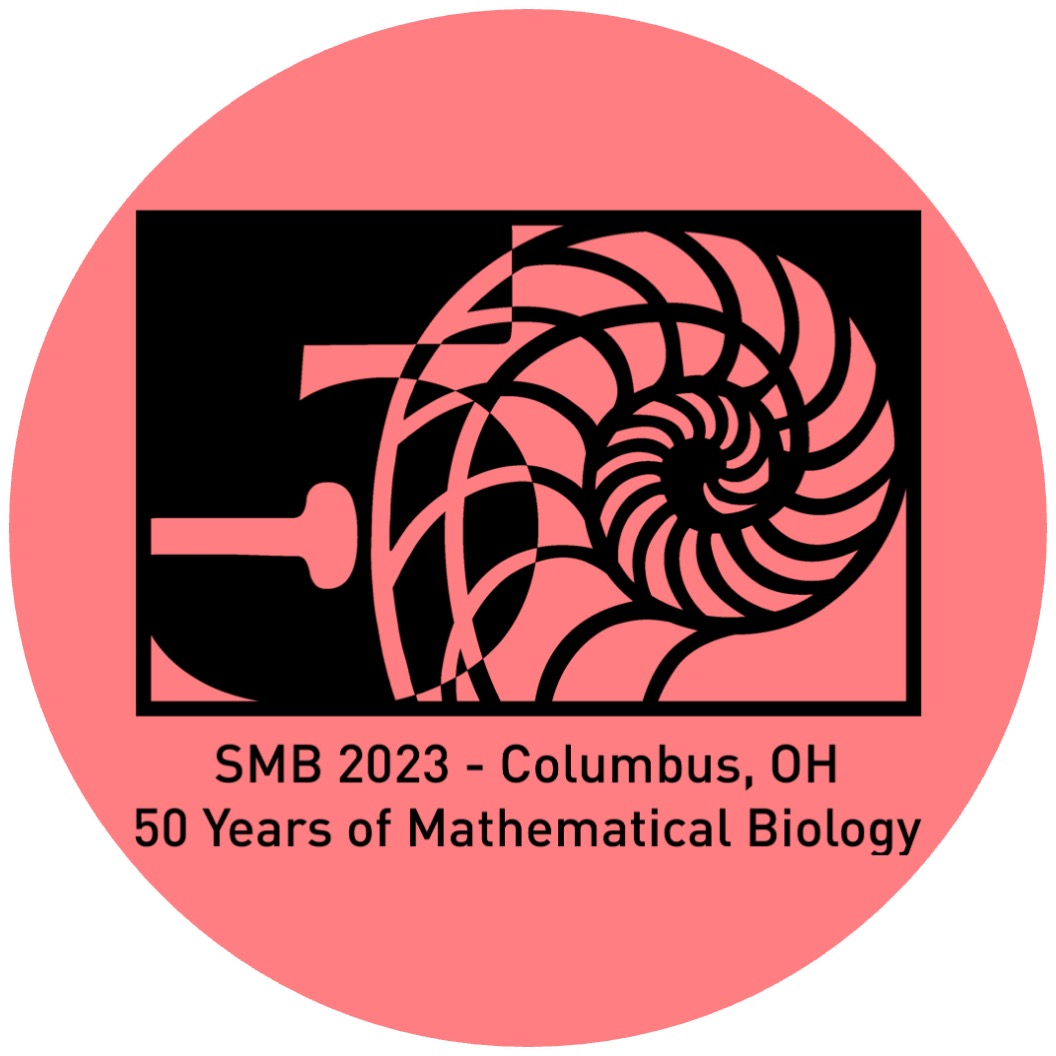
Organizers:
Celeste Vallejo
Description:
Preparing for the next pandemic is going to require cooperation and coordination amongst many groups of people. One such group is those working in drug development. Drug development is a crucial piece of the puzzle in preparing for the next pandemic. This is exemplified in the effect that the COVID-19 vaccine had on the outcome of this most recent pandemic. In this mini-symposium, speakers from across the drug development space are coming together to describe various ways in which modeling and simulation in drug development can be used to prepare for the next pandemic. The goal of this session is to inform the mathematical biology community, especially those that have or continue to work with government officials, on how those in the drug development space are thinking about problems related to drug development, what tools are being used, and the contributions that can be made towards preparing for the next pandemic.
Sriram Chandrasekaran
University of Michigan (Biomedical Engineering)"Drug discovery and repurposing using hybrid machine learning and biochemical modeling"
Amber Smith
University of Tennessee Health Science Center (Department of Pediatrics)"PKPD modeling of Plasmodium falciparum ATP4 inhibitor SJ733 with the pharmacokinetic enhancer cobicistat"
Celeste Vallejo
Simulations Plus, Inc. (DILIsym Services)"Potential application of a mechanistic model of chronic lung disease to the treatment of post-COVID lung fibrosis and other respiratory pandemics"
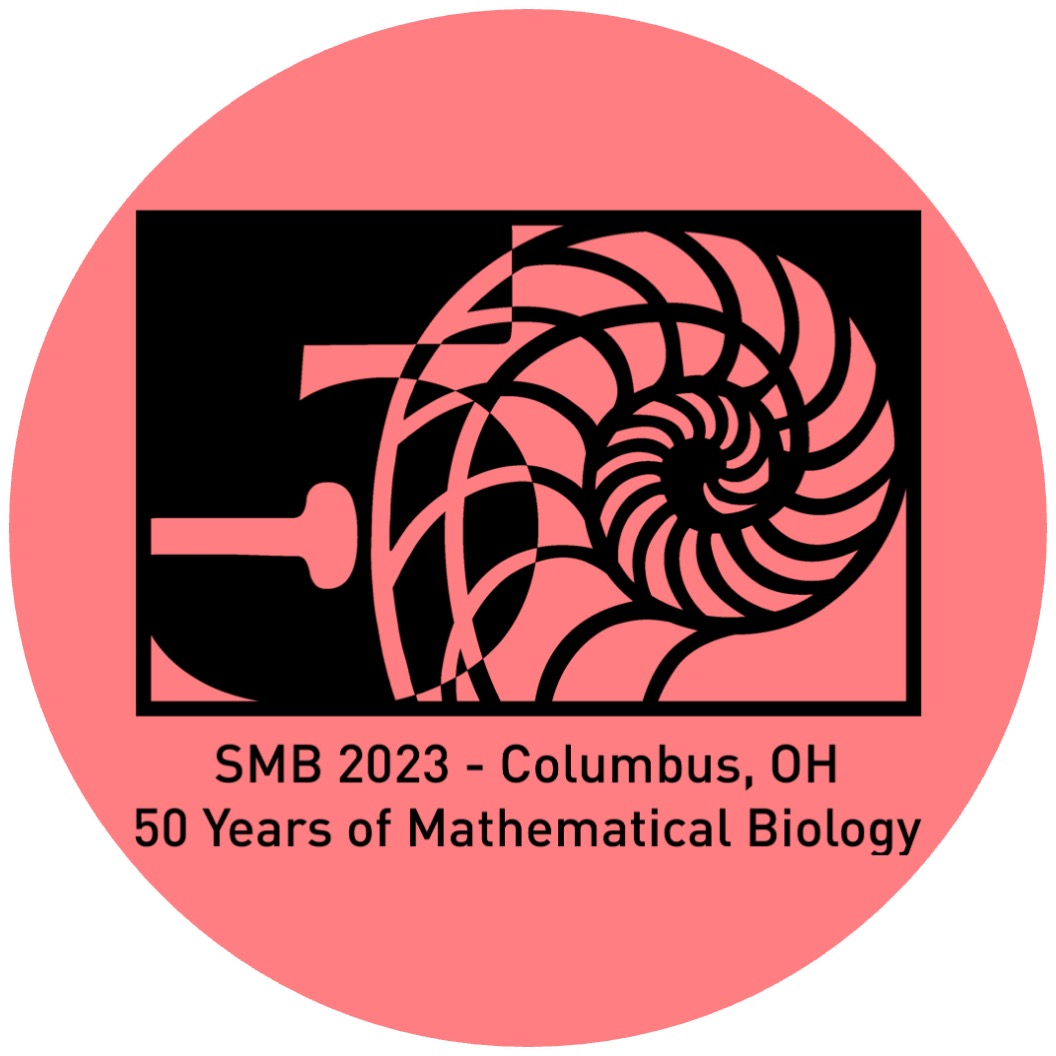