MS05 - ONCO-1 Ohio Staters Traditions Room (#2120) in The Ohio Union
Digital twins for clinical oncology and cancer research
Wednesday, July 19 at 10:30am
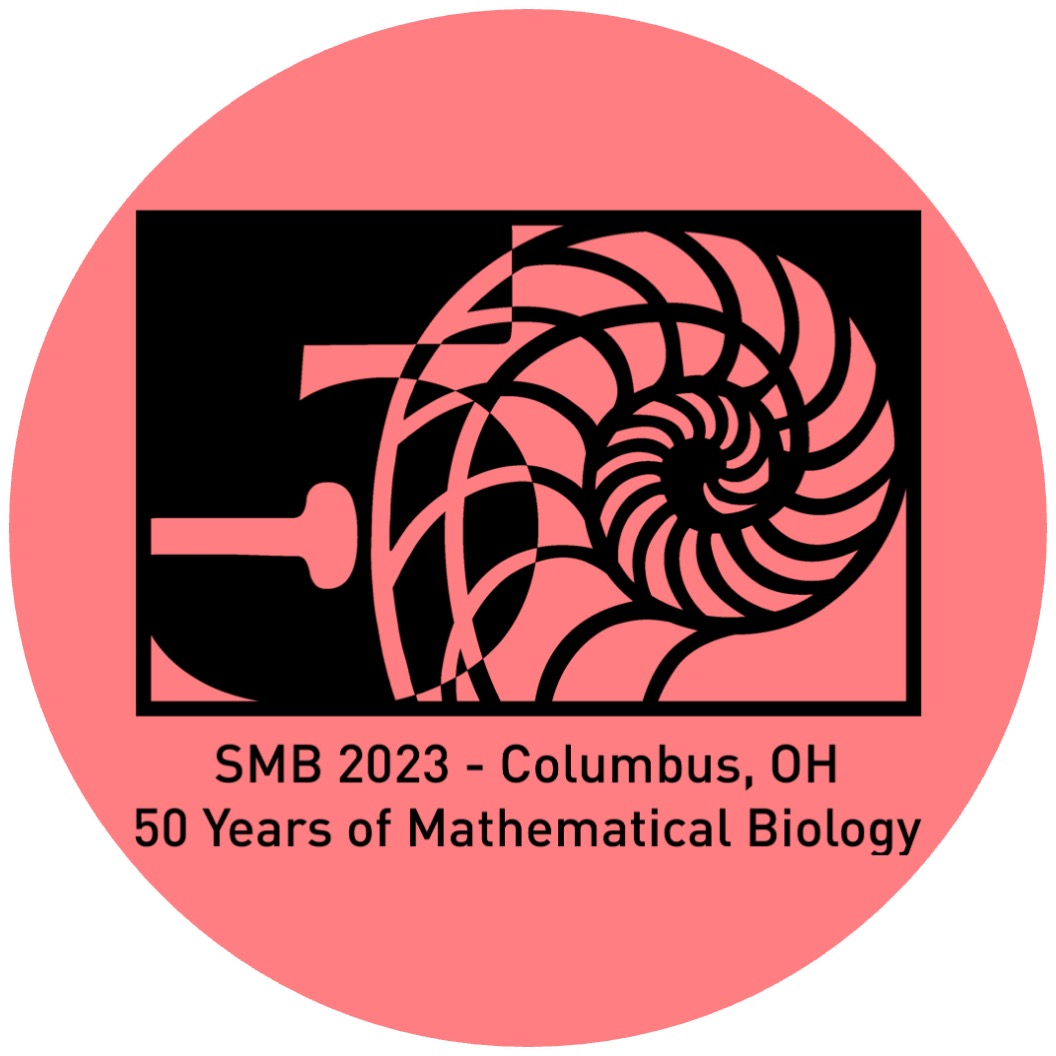
Organizers:
Guillermo Lorenzo, Chengyue Wu, David A Hormuth II, Ernesto A. B. F. Lima, Lois C. Okereke, Thomas E. Yankeelov
Description:
The overall goal of this minisymposium is to present and discuss recent developments of digital twin technologies to (i) address the personalization and optimization of the clinical management of cancers, and (ii) advance the research of the biophysical mechanisms underlying these pathologies from the micro to the macroscale. A digital twin can be defined as a virtual representation of a physical object by means of a computational model (or a collection of models) that can continuously assimilate object-specific data to enable-decision making about the physical object based on its current and future states. Digital twins have undergone a widespread development in multiple areas of engineering (e.g., design, fabrication, and health monitoring of industrial products; energy and industrial plant operation; automatization of industrial components). Additionally, digital twins have been proven useful in several areas of medicine, such as surgical planning, cardiovascular disease interventions, convection-enhanced delivery of drugs to the brain, or glucose monitoring in diabetic patients. Given the increasing success of computational models to predict the development of cancer and its response to treatments, these models could be employed to construct digital twins to support the optimal diagnosis, monitoring, and treatment of individual patients as well as to assist the research of this disease in vitro, in vivo, and in silico. The talks in this minisymposium will present recent efforts in building digital twins for the clinical management of cancers and the (pre)clinical research of these diseases. The presentations will include a description of the specific area of application of the digital twins, the underlying models and computational techniques, the types of data required for their operation, performance metrics, and future developments.
Stéphane Bordas
University of Luxembourg (Department of Engineering Sciences)"Digital twinning physiological processes: brain metabolism and cancer growth"
Jesús J. Bosque
University of Castilla-La Mancha (Spain) (Mathematical Oncology Laboratory (MOLAB))"Less is more in glioma treatment: In silico and in vivo evidence towards a clinical trial"
Renee Brady-Nicholls
H. Lee Moffitt Cancer Center & Research Institute (Integrated Mathematical Oncology)"An In Silico Study of Hormone Therapy in Metastatic Prostate Cancer"
Chase Christenson
University of Texas at Austin (Biomedical Enginering)"Fast digital twin construction for modeling the response of breast cancer to therapy using proper orthogonal decomposition."
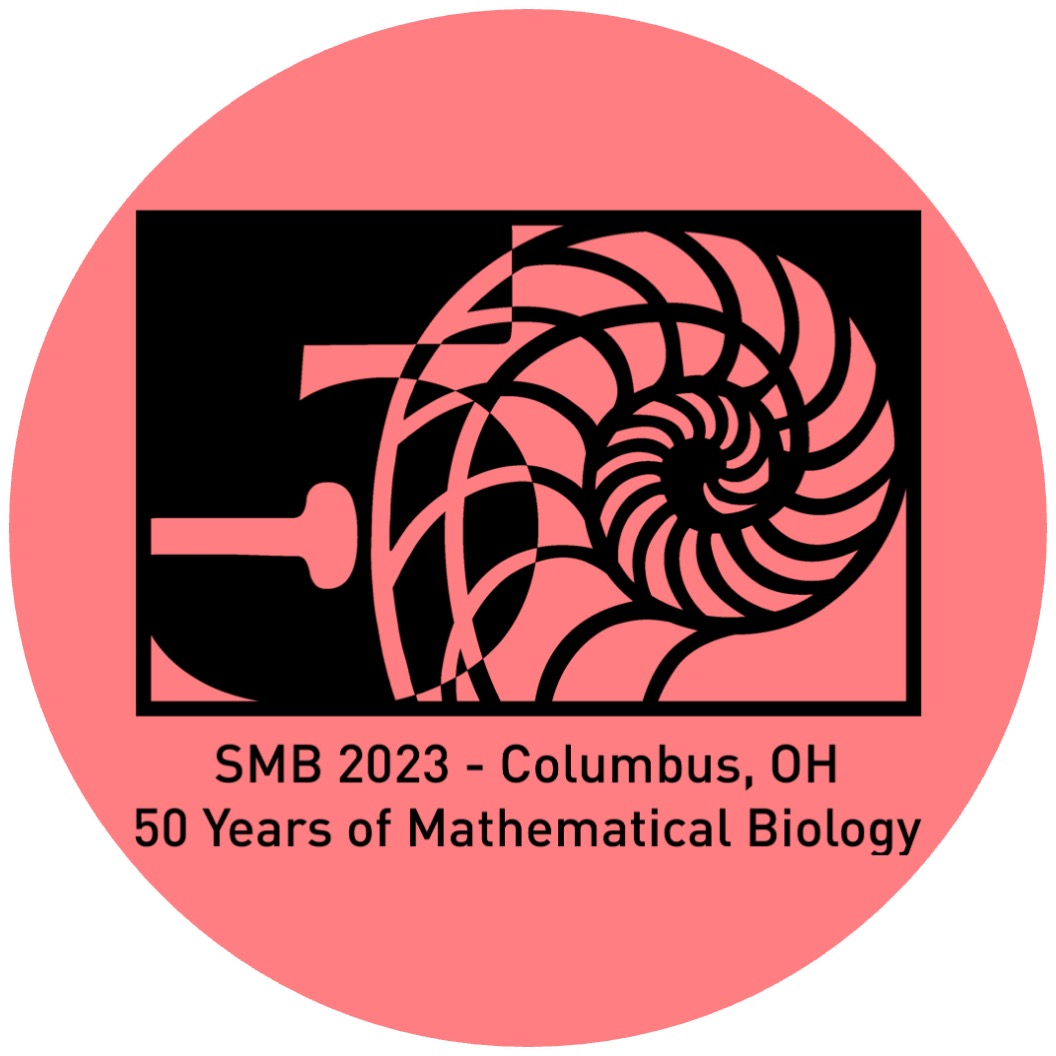