CT01 - ONCO-2 Griffin East Ballroom (#2133) in The Ohio Union
ONCO Subgroup Contributed Talks
Monday, July 17 at 2:30pm
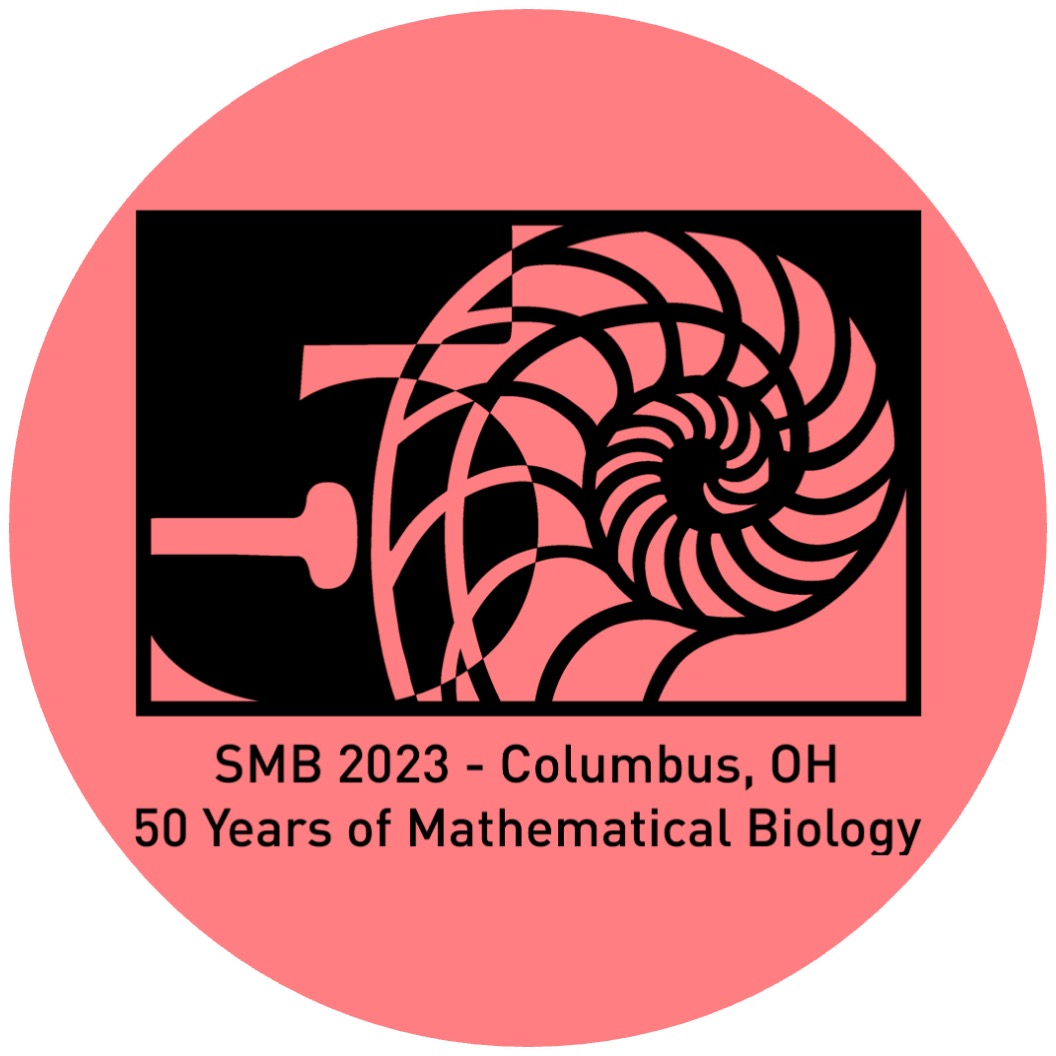
Share this
Chloe Colson
University of Oxford"Investigating the impact of growth arrest mechanisms on tumour responses to combinations of radiotherapy and hyperthermia"
Louis Kunz
Massachusetts General Hospital and Harvard Medical School"AMBER (Agent-based Modeling of Biophysical Evolution after Radiotherapy): a computational tumor model as the first step to simulate radiation response."
Noemi Andor
Moffitt Cancer Center"Modeling competition between subpopulations with variable DNA content in resource limited microenvironments"
Paul Macklin
Indiana University"A new grammar for real-time and reproducible modeling of multicellular interactions in cancer"
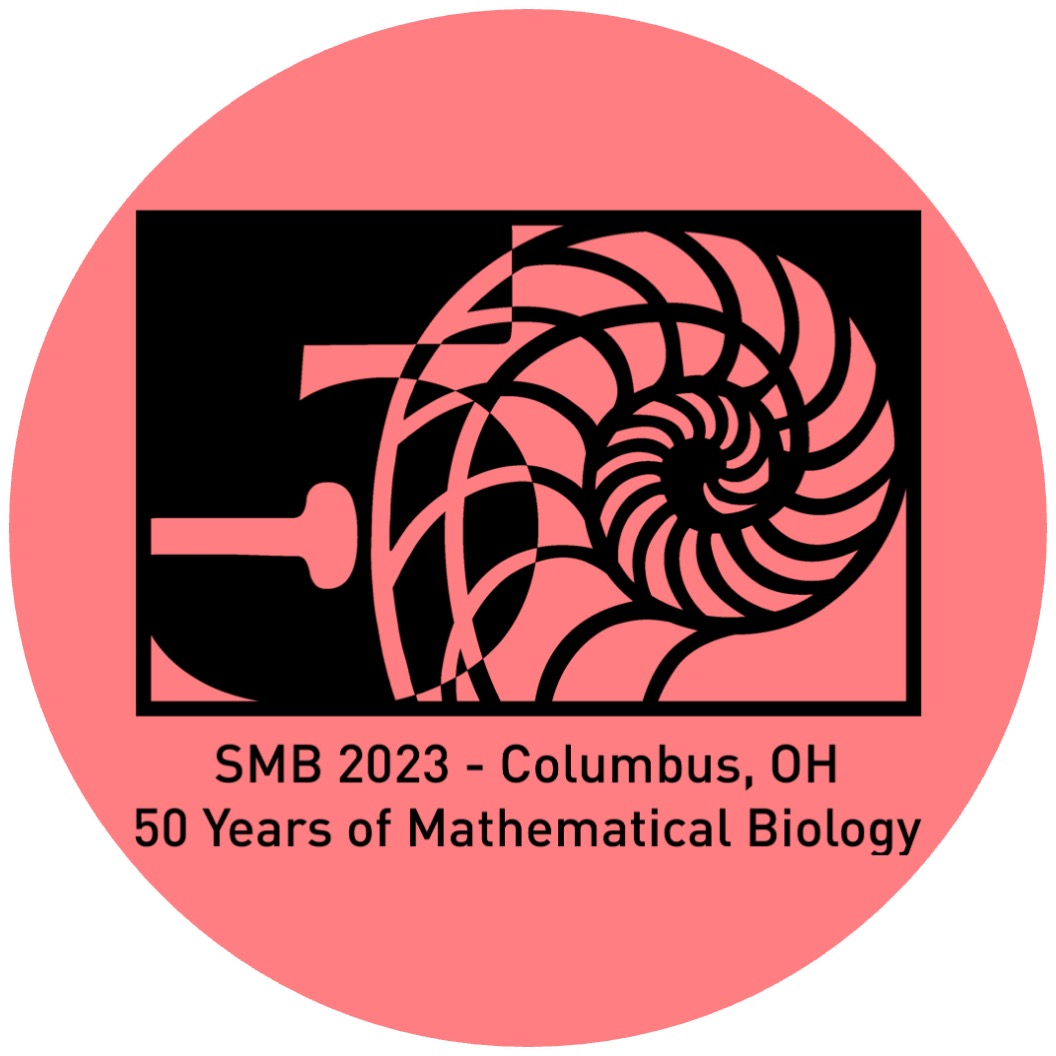