MS08 - ONCO-1 Ohio Staters Traditions Room (#2120) in The Ohio Union
Emerging Leaders in Mathematical Oncology: The MathOnco Subgroup Minisymposium
Friday, July 21 at 10:30am
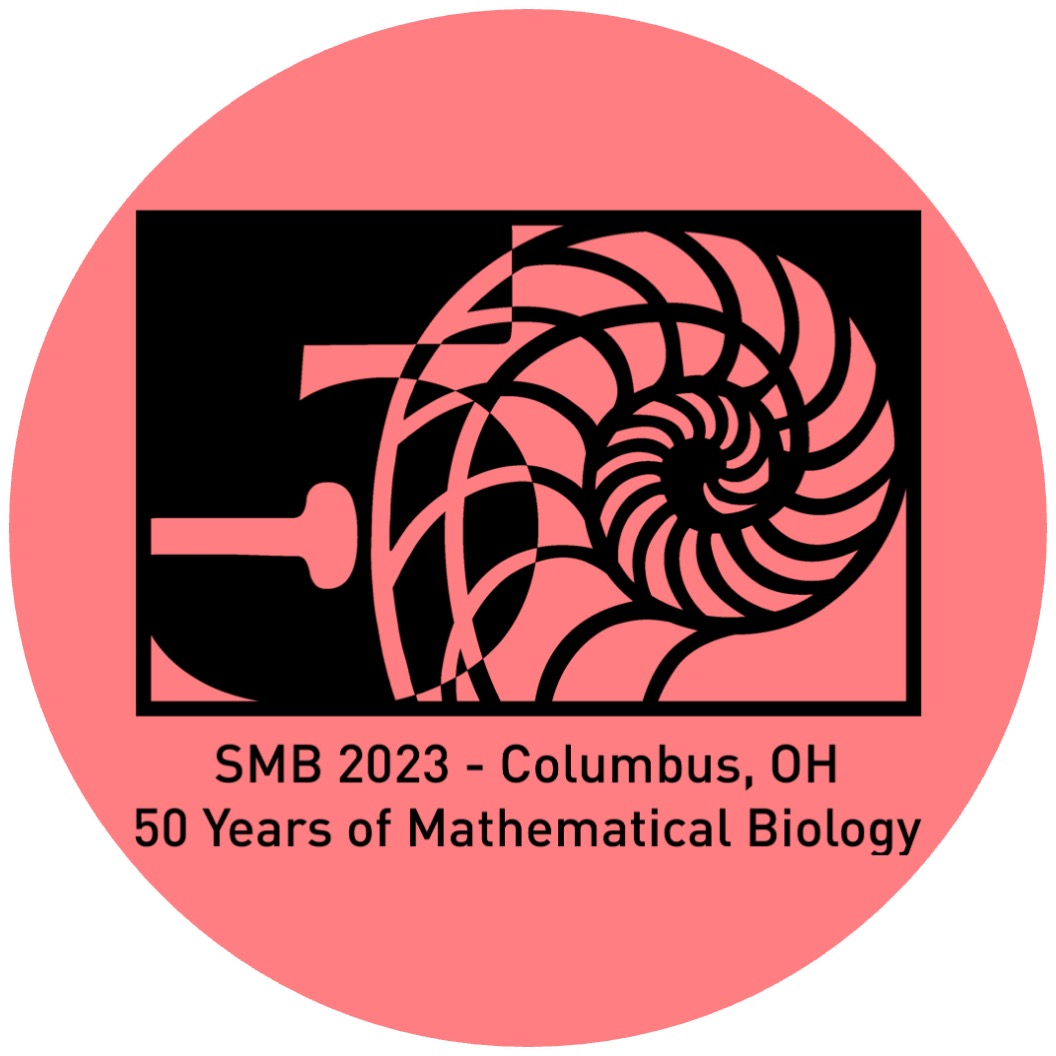
Organizers:
Renee Brady-Nicholls, Harsh Jain, Jason George
Description:
Mathematical oncology continues to emerge as an important area of research focused on understanding the complexity and intricacies of cancer using mathematical modeling. New methodologies and techniques are constantly being developed to better understand and predict how the disease evolves and adapts to treatment. This minisymposium will feature talks from emerging leaders in mathematical oncology – specifically graduate students, postdocs, and early-stage independent researchers. These rising stars will highlight new and innovative contributions to mathematical oncology research using differential equation models, ABMs, and multi-scale models.
Maximilian Strobl
Cleveland Clinic (Department for Translational Hematology and Oncology Research, Lerner Research Institute)"Using mathematical modeling to design protocols for preclinical testing of evolutionary therapies"
Rebecca A. Bekker
H. Lee Moffitt Cancer Center & Research Institute (Department of Integrated Mathematical Oncology)"The Immunological Consequences of Spatially Fractionated Radiotherapy"
Ibrahim Chamseddine
Massachusetts General Hospital, Harvard Medical School (Radiation Oncology)"Towards Personalized Oncology: Machine Learning-Driven Radiotherapy Across Multiple Disease Sites"
Alexander B. Brummer
College of Charleston (Department of Physics and Astronomy)"Data-driven model discovery and interpretation for CAR T-cell killing using sparse identification and latent variables"
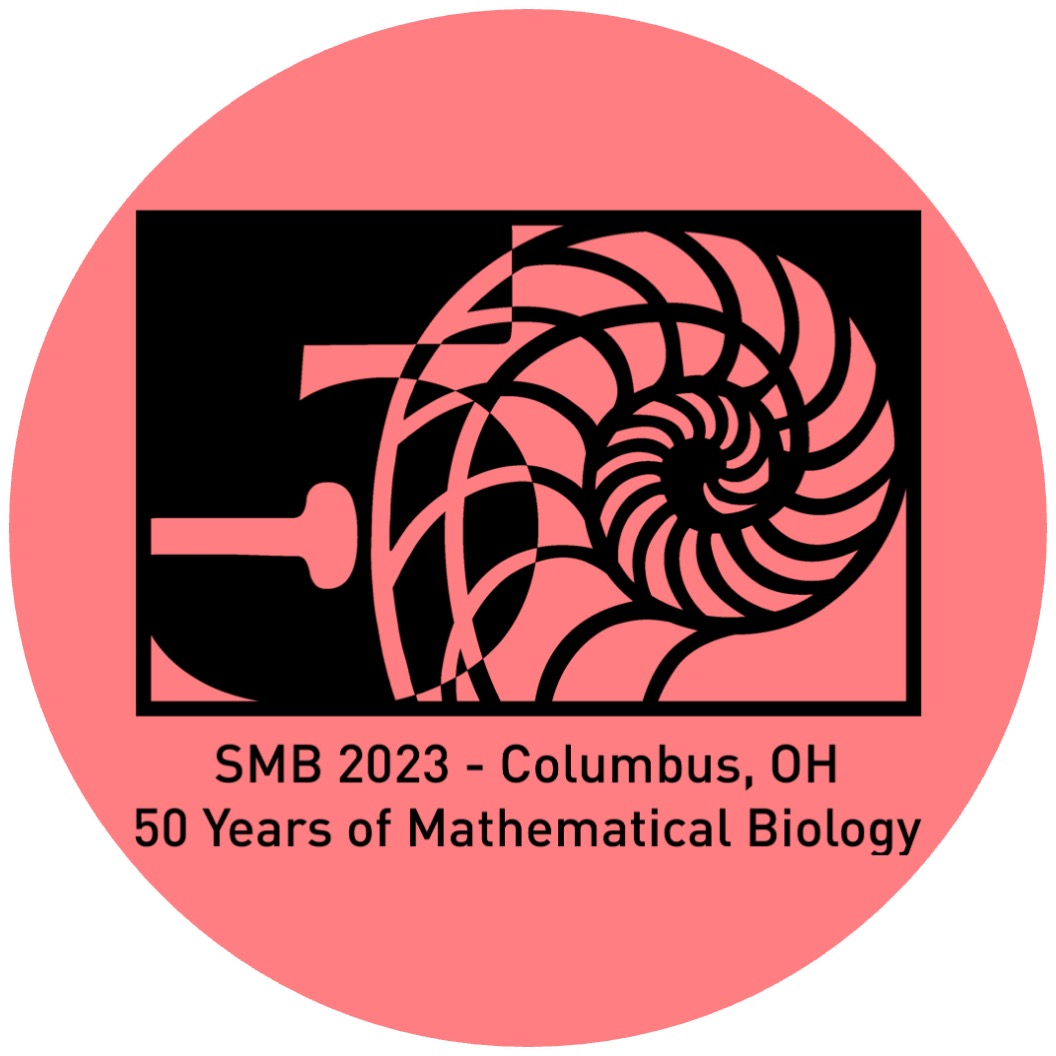