MS02 - ONCO-1 Ohio Staters Traditions Room (#2120) in The Ohio Union
Techniques and Methods in Modelling Cancer Treatment
Monday, July 17 at 04:00pm
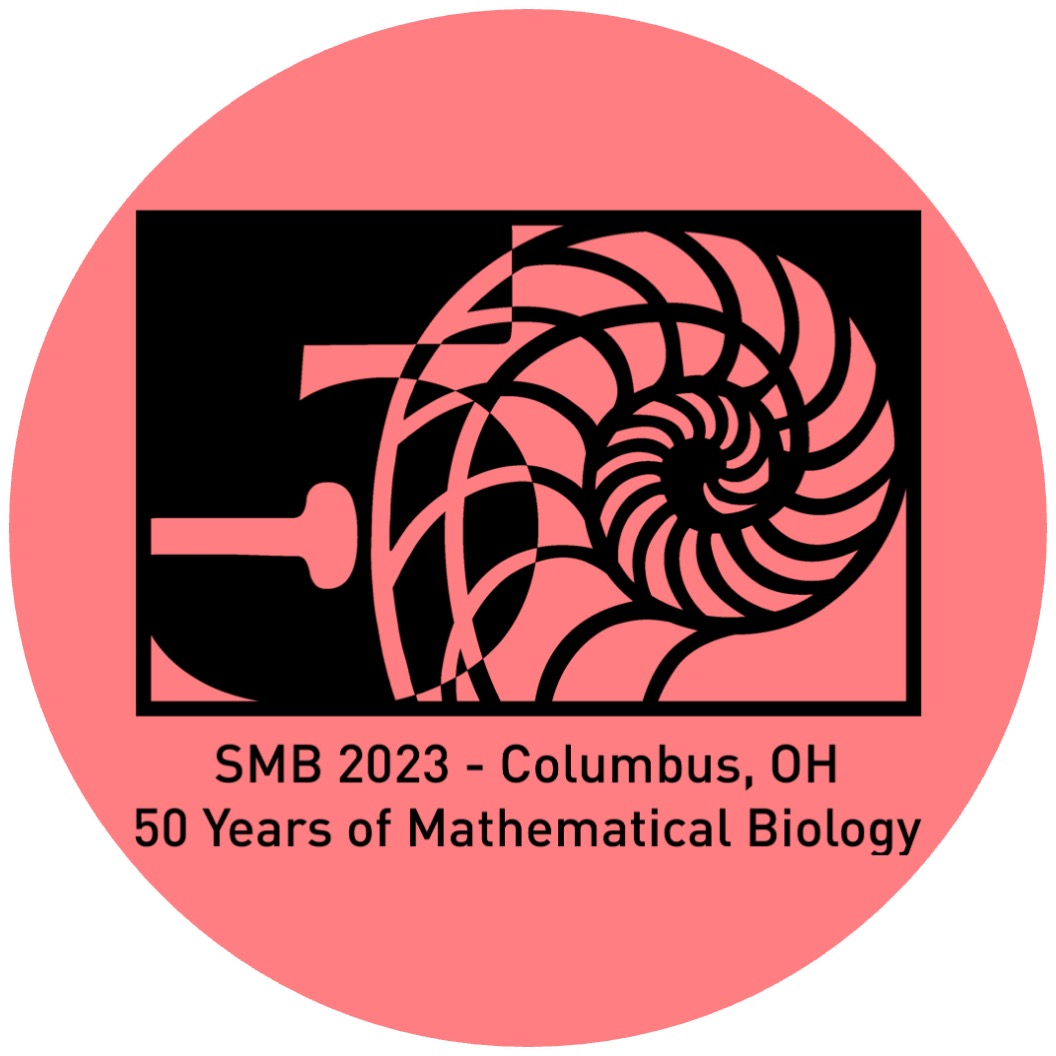
Organizers:
Kathleen Wilke, Gibin Powathil
Description:
This mini-symposium aims to bring together speakers that will highlight advances in the various mathematical methods and techniques used in the study of cancer treatment. There are numerous aspects to consider in the biological response of a treated tumour within the human body. Here we will highlight many of these factors, and the diverse modelling approaches needed to describe and analyse the motivating complex system. Talks will be of a great interest to a wide range of participants due to the variety of topics such as radiation, targeted therapy, and immunotherapy, and due to the variety of modelling approaches and techniques such as ODES, Fractional-DEs, PDEs, PK-PD, multi scale, and systems modelling.
Annabelle Ballesta
Inserm & Institut Curie (unit 900)"Quantitative Systems Pharmacology to Personalize Temozolomide-based Drug Combinations against Brain Tumors."
Kévin Spinicci
Swansea University"Mathematical modelling of HIF on regulating cancer cells metabolism and migration"
Linh Nguyen Phuong
Aix-Marseille University (COMPutational pharmacology and clinical Oncology Team)"Mechanistic modeling of the longitudinal tumor and biological markers combined with quantitative cell-free DNA"
Heiko Enderling
Moffitt Cancer Center (Department of Integrated Mathematical Oncology)"Mathematical modeling of cancer radiotherapy"
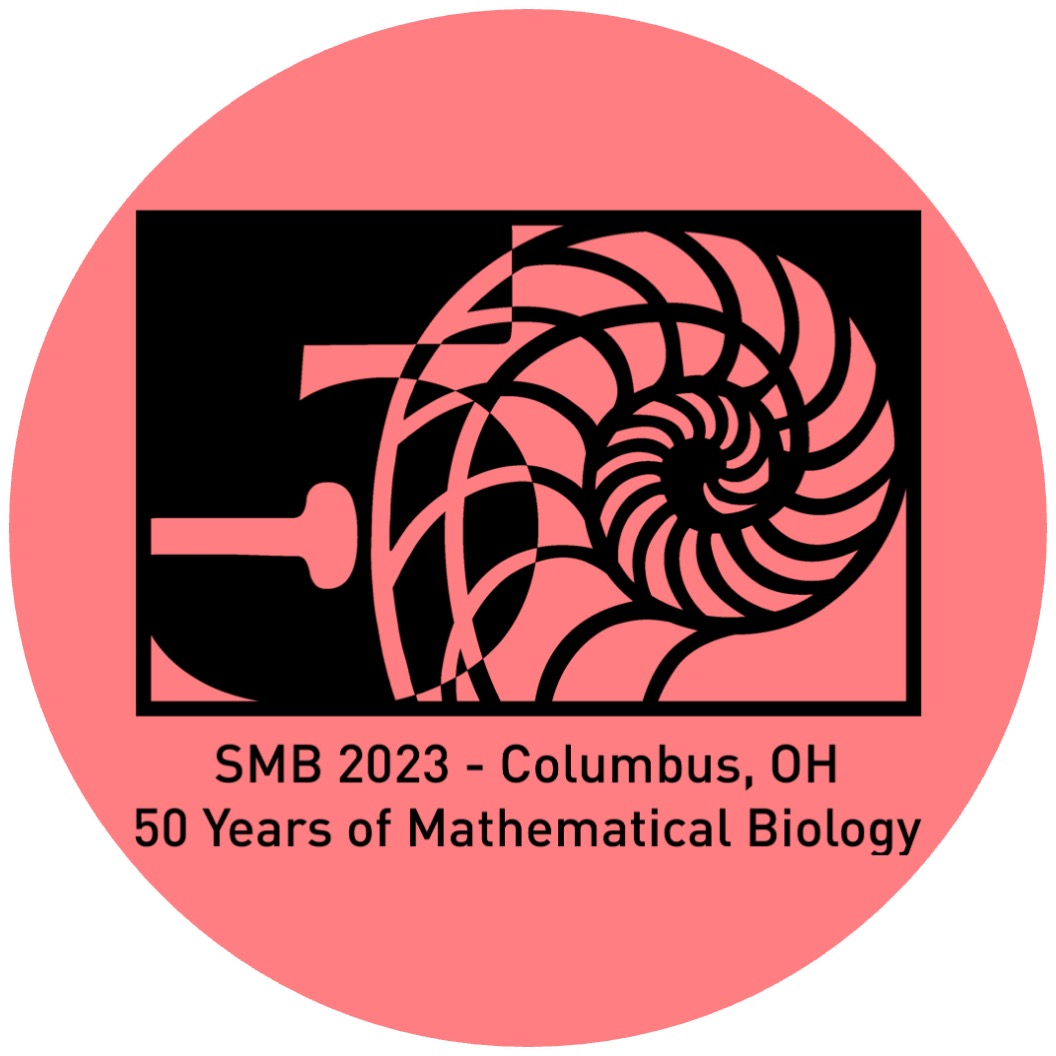