MS02 - IMMU-1 Brutus Buckeye Room (#3044) in The Ohio Union
Multiscale Mechanistic Modeling in Immunology (in celebration of Denise Kirschner’s 60th birthday)
Monday, July 17 at 04:00pm
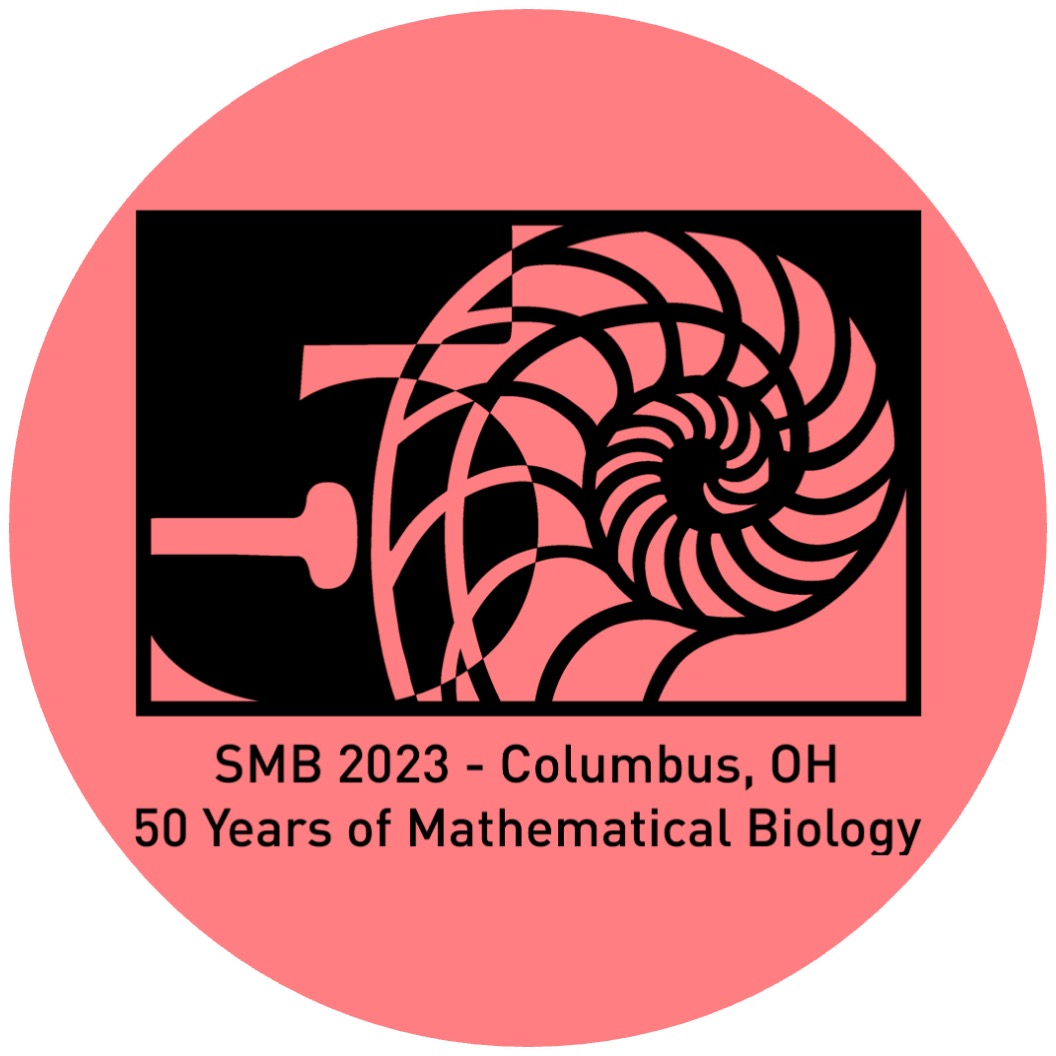
Organizers:
Marissa Renardy, Caitlin Hult
Description:
Denise Kirschner is a pioneering woman in mathematical biology, a strong mentor to numerous graduate students and postdocs, and is a past president (2017-2019) and long-time member of SMB. This minisymposium is in celebration of Denise Kirschner's 60th birthday and consists of talks by her current and former postdocs. The topic focuses on multi-scale and mechanistic modeling in immunobiology and infection, with applications including oncology, tuberculosis, and non-tuberculous mycobacterial infections. Speakers from industry and academia will highlight the importance of mathematical modeling in optimizing treatment regimens, interpreting imaging data, and understanding disease progression, among other topics.
Marissa Renardy
Applied BioMath (Modeling)"Capturing CAR-T cell therapy dynamics through semi-mechanistic modeling"
Pariksheet Nanda
University of Michigan Medical School (Microbiology and Immunology)"Calibrating multivariate models using CaliPro and approximate Bayesian computing"
Maral Budak
University of Michigan Medical School (Department of Microbiology & Immunology)"Optimizing regimen treatment during the host-pathogen interaction of tuberculosis using a multi-scale computational model"
Christian Michael
University of Michigan - Michigan Medicine (Microbiology & Immunology)"Towards digital partners of Mycobacterium tuberculosis infection within a virtual city framework"
Elsje Pienaar
Purdue University (Biomedical Engineering)"Biofilm impacts in Non-tuberculous mycobacterial infections in the airway"
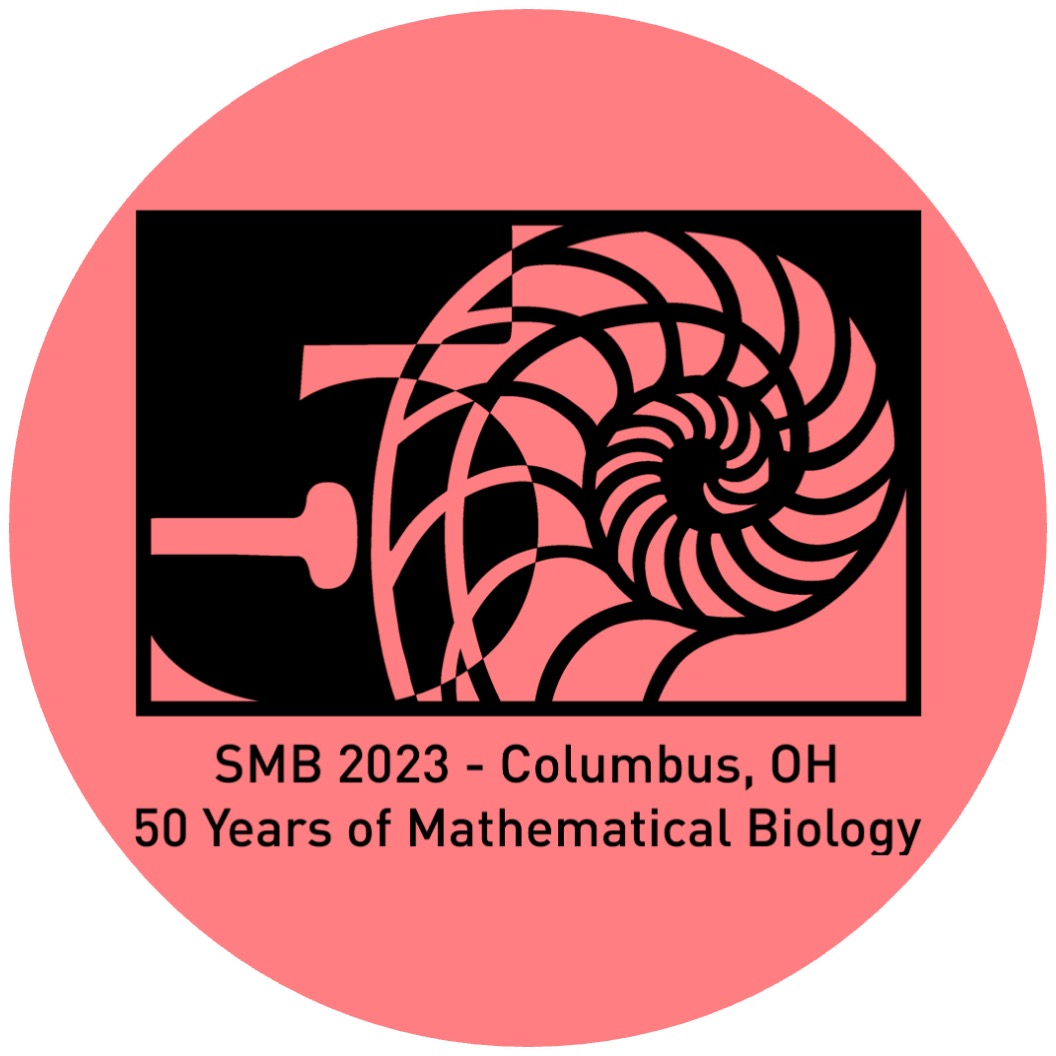