MS06 - MFBM-2 Suzanne M. Scharer Room (#3146) in The Ohio Union
Data-driven multiscale modeling of cancer
Thursday, July 20 at 10:30am
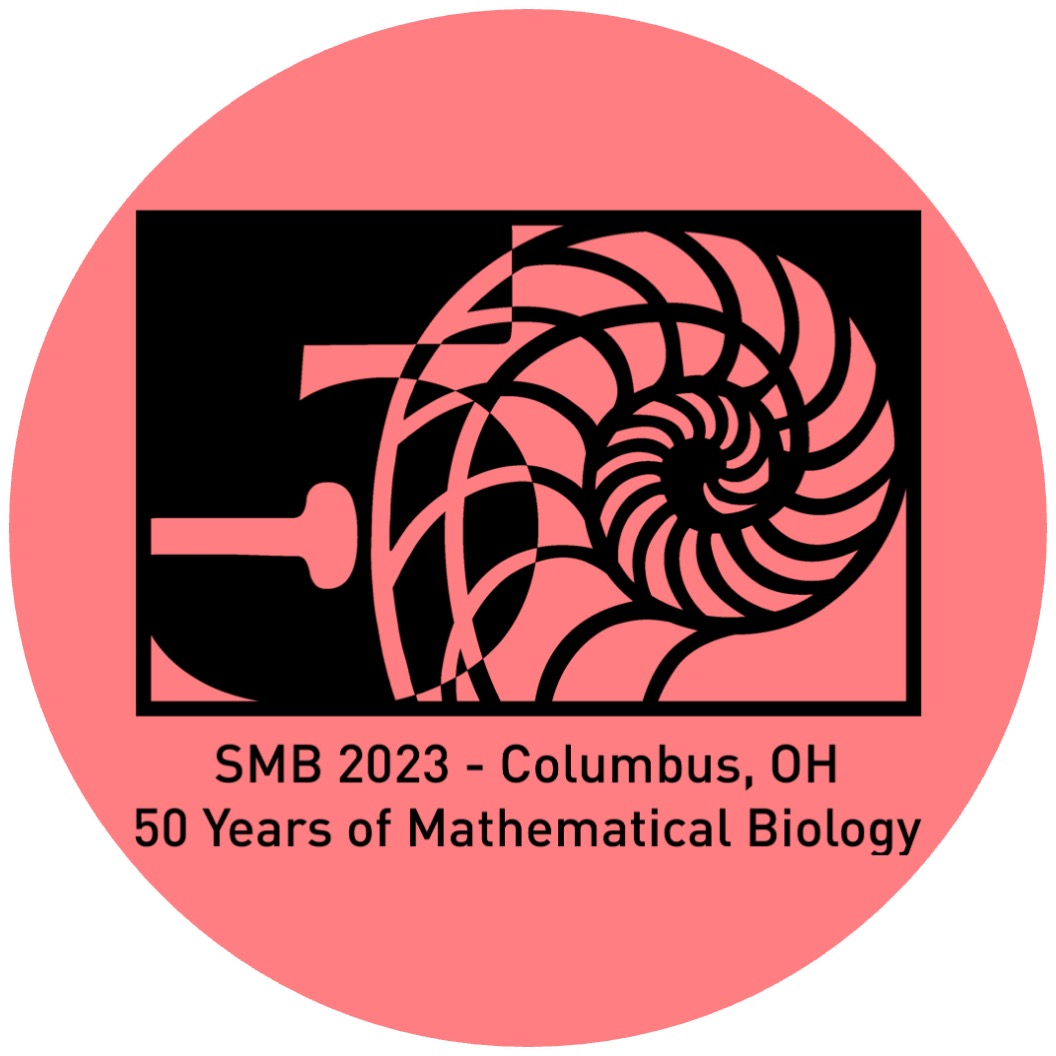
Organizers:
Heber Rocha, John Metzcar, Paul Macklin
Description:
The integration of data, such as multiplexed -omics data, histopathology slides, or cell viability and motility assays, into multiscale simulations is a complex and challenging problem. The available data is often high-dimensional, noisy, sparse, and variable in both time and space. On the modeling side, there are many parameters to consider, and numerous possible emergent outcomes may arise. The first step in this process is to map the data to model parameters. This is often a difficult task due to the complexity of the data and the large number of parameters that must be considered. Once the data has been mapped to the model parameters, it is crucial to confirm that the model can reproduce the observations with appropriate fidelity. This step is critical to ensuring that the model is accurate and reliable. In this mini-symposium, we explore new and emerging techniques to address these challenges. We examine the integration of qualitative and quantitative data at each level of the modeling process. Additionally, we discuss the impacts of parameter uncertainty in the quality of model predictions. Overall, this mini-symposium focuses on the challenges of integrating data into multiscale simulations and the techniques and strategies that can be used to overcome these challenges.
Alexander Browning
University of Oxford (Mathematical Institute)"Drawing biological insight from non-identifiabile models of tumour growth using simple surrogates"
Jeanette Johnson
Johns Hopkins University (Immunology)"Integrating Omics Data and Agent-Based Models for Comprehensive Digital Biology"
Adam MacLean
University of Southern California (Department of Quantitative and Computational Biology)"Learning gene regulatory networks that control cell state transitions from multi-modal single-cell genomics"
Matthew Simpson
Queensland University of Technology (School of Mathematical Sciences)"A stochastic mathematical model of 4D tumour spheroids with real-time fluorescent cell cycle labelling"
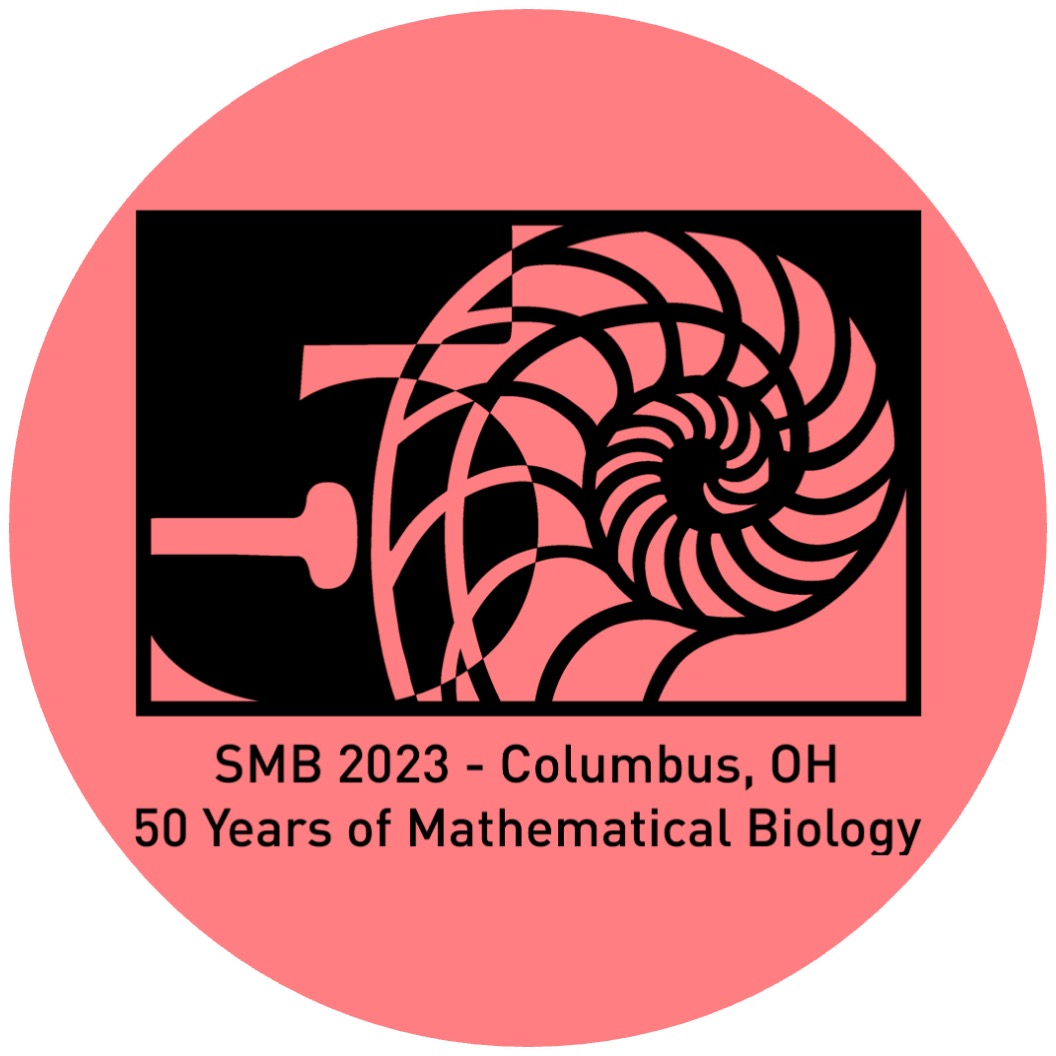