MS03 -
MFBM-2
Griffin West Ballroom (#2133) in The Ohio Union
Inference, analysis, and control of Boolean network models
Tuesday, July 18 at 10:30am
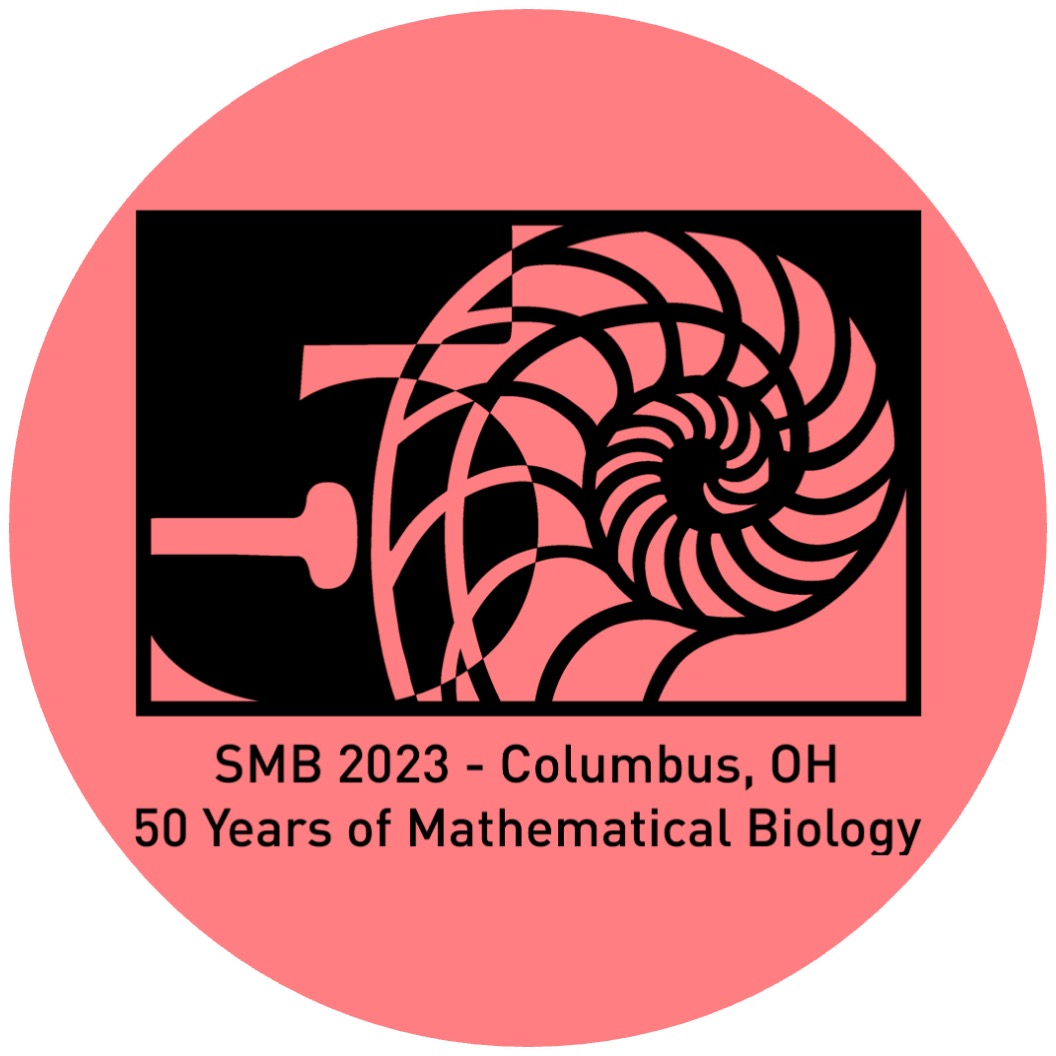
Room assignment: Griffin West Ballroom (#2133) in The Ohio Union.
Organizers:
David Murrugarra
Description:
Boolean networks are becoming increasingly popular models and have been successfully used for modeling important gene regulatory networks. This mini symposium will highlight challenges and new developments in processes such as model building, model analysis, and control. This session will cover methods for reverse engineering of Boolean networks from data, canalization, modularity, and phenotype control. This minisymposium will offer a sequential view of the process of going from data to obtain a model, then to analyze the model to validate it, and finally to control the model to obtain intervention targets.
Elena Dimitrova
California Polytechinc State University, San Luis Obispo (Mathematics)"A unified approach to reverse engineering and data selection for unique network identification"
Brandilyn Stigler
Southern Methodist University (Mathematics)"Computational Algebraic Methods for Boolean Network Modeling"
Claus Kadelka
Iowa State University (Department of Mathematics)"A modular design of gene regulatory networks emerges naturally in response to an evolutionary multi-objective optimization problem"
Daniel R. Plaugher
University of Kentucky (Department of Toxicology and Cancer Biology)"Phenotype control techniques for gene regulatory networks"
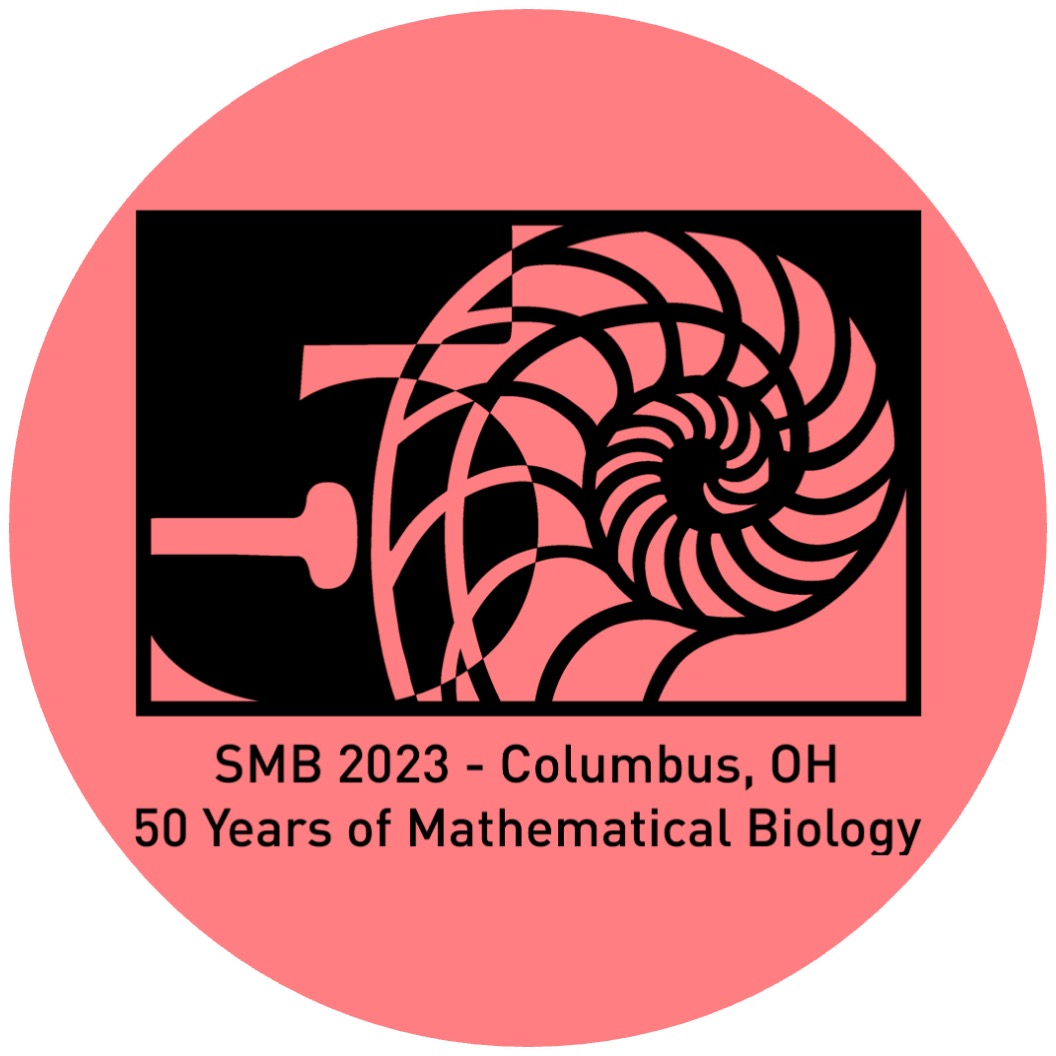