MS01 - MFBM-2 Griffin West Ballroom (#2133) in The Ohio Union
Integration of data and modeling for multiscale biology
Monday, July 17 at 10:30am
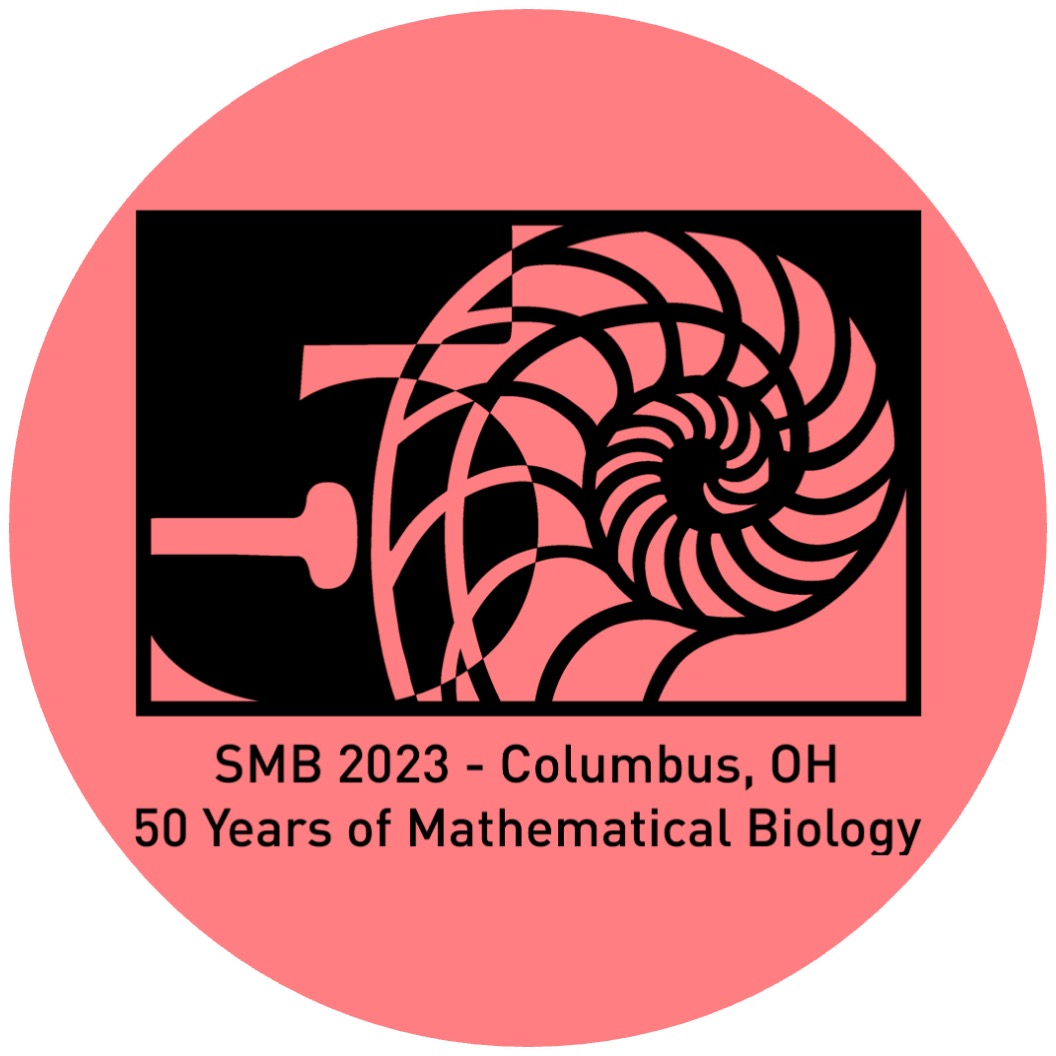
Organizers:
Yuchi Qiu, Heyrim Cho
Description:
Breakthroughs in biotechnology have enabled quantitative interrogation of biological mechanisms, and the resulting large-scale datasets present unique opportunities and challenges for understanding the spatial-temporal dynamics of biological systems. This mini-symposium aims to bring researchers together to discuss the latest advanced data-driven and multiscale modeling methodologies for complex biological systems that bridge gene, cellular, and tissue morphogenetic dynamics and their interactions. The topics will explore cutting-edge methodologies in artificial intelligence, optimal transport, dynamical systems, stochastic modeling, and more, emphasizing on cell and cancer biology. Researchers will have the opportunity to share their research findings and collaborate to gain a deeper understanding of the underlying mechanisms in cell fate decisions and cancer progression.
Jianhua Xing
University of Pittsburgh (Computational and Systems Biology)"Reconstructing cellular dynamics from single cell data"
Aden Forrow
University of Maine (Mathematics and Statistics)"Trajectory inference with lineage tracing"
Yuchi Qiu
Michigan State University (Department of Mathematics)"Interpretable AIs in data-driven biology: from topological data analysis to multiscale modeling"
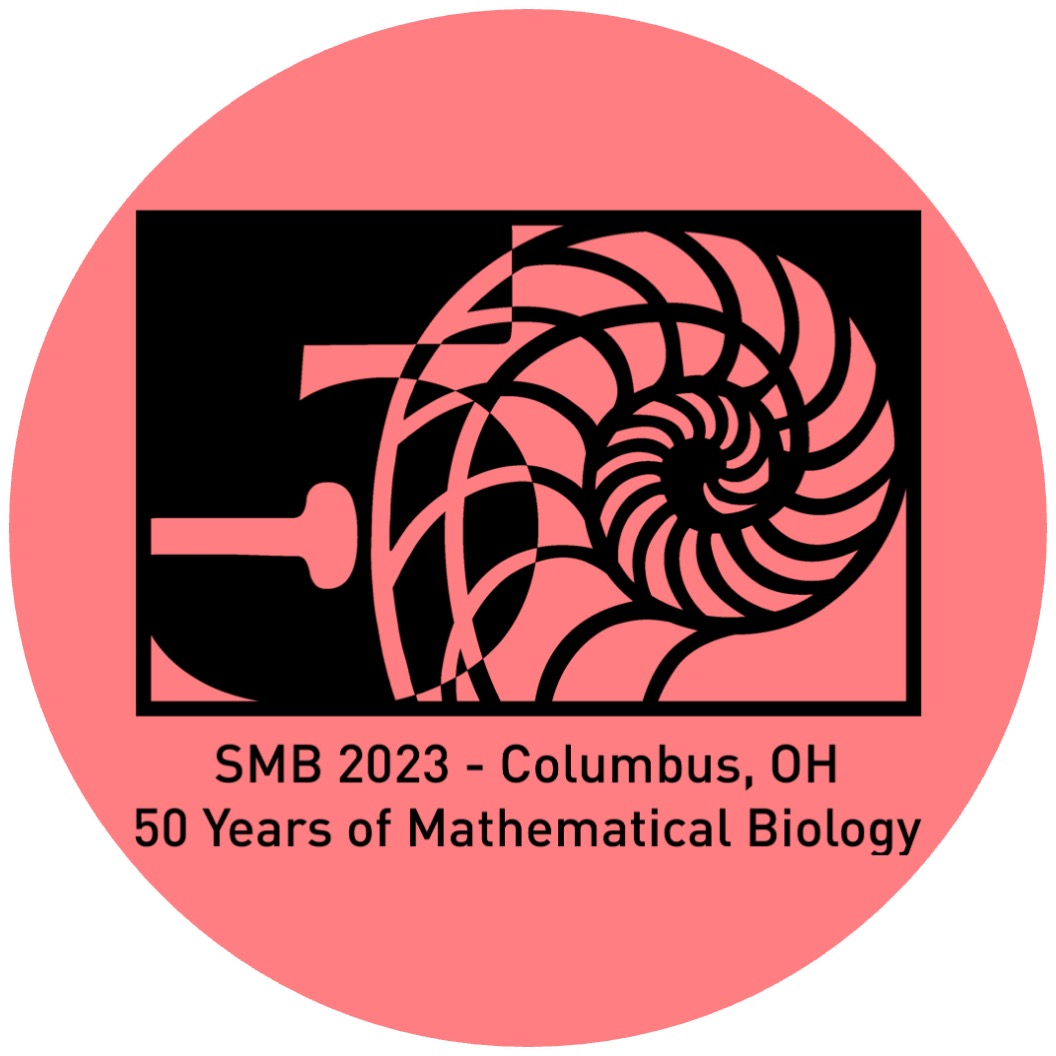