MS06 - MEPI-1 Cartoon Room 1 (#3145) in The Ohio Union
Recent advances in parameter identifiability of mathematical models in mathematical biology
Thursday, July 20 at 10:30am
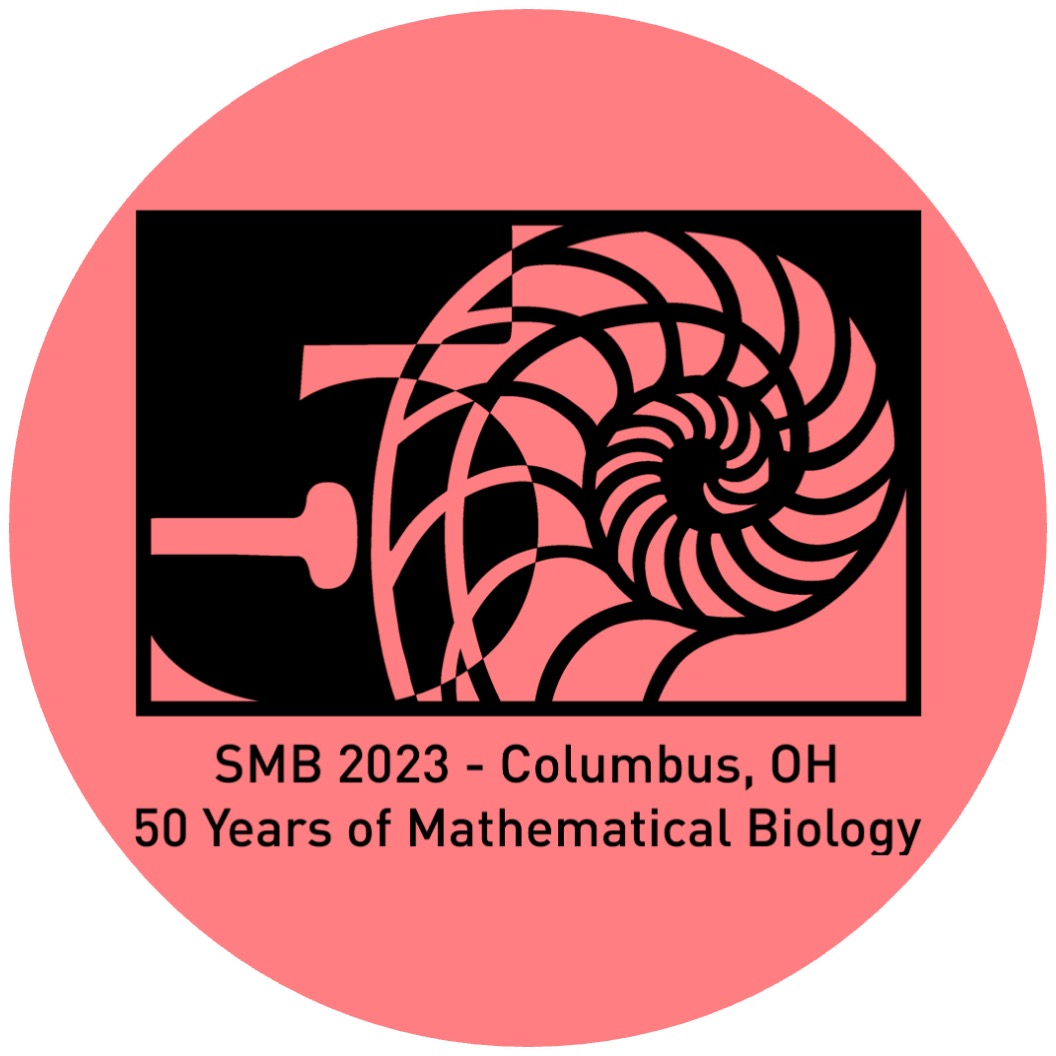
Organizers:
Omar Saucedo, Bren Case, Lauren Childs
Description:
Parameter estimation is common practice when determining unknown parameters of a mathematical model. However, whether estimated parameters are reliable depends on the quantity and quality of available data, as well as the complexity of the model. Although models in mathematical biology tend to be highly nonlinear and can interact with data in unexpected ways, identifiability is rarely assessed prior to model fitting. Identifiability analysis comes in two flavors, the first focusing on whether there exists a unique set of parameters for all possible model output, and the second focusing the set of plausible parameters inferred from noisy data. Both approaches employ a range of geometric and statistical techniques. The goal of this mini-symposium is to bring in experts working throughout the immense field of mathematical biology to discuss the most recent advancements in identifiability.
Widodo Samyono
Jarvis Christian University (Mathematics and Sciences)"Parameters Identifiability for selecting the best model using differential equations optimization"
Marisa Eisenberg
University of Michigan, Ann Arbor (Epidemiology and Complex Systems)"Identifiability and infectious disease interventions: exploring when uncertainty matters"
All Participants
"Open Forum"
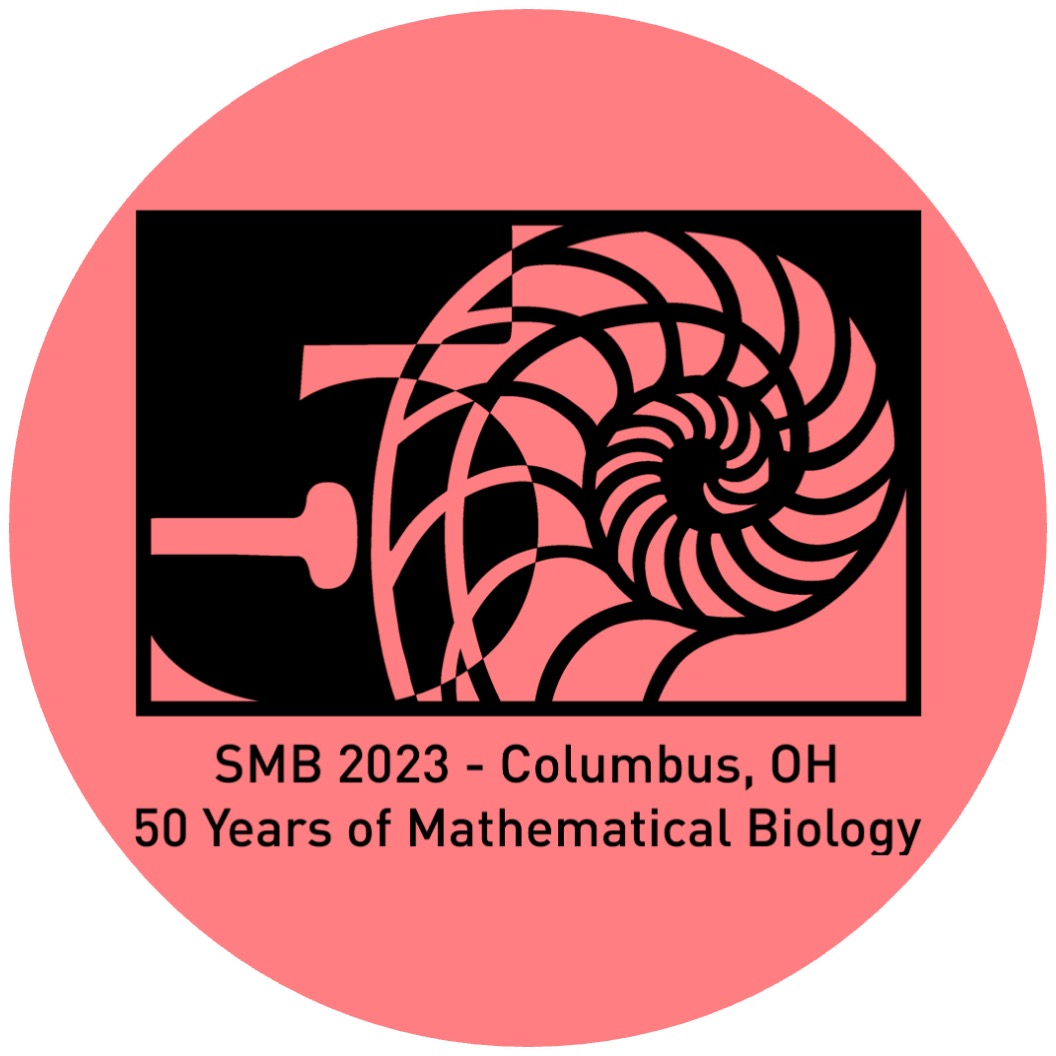