CT01 - IMMU-2 Cartoon Room 2 (#3147) in The Ohio Union
IMMU Subgroup Contributed Talks
Monday, July 17 at 2:30pm
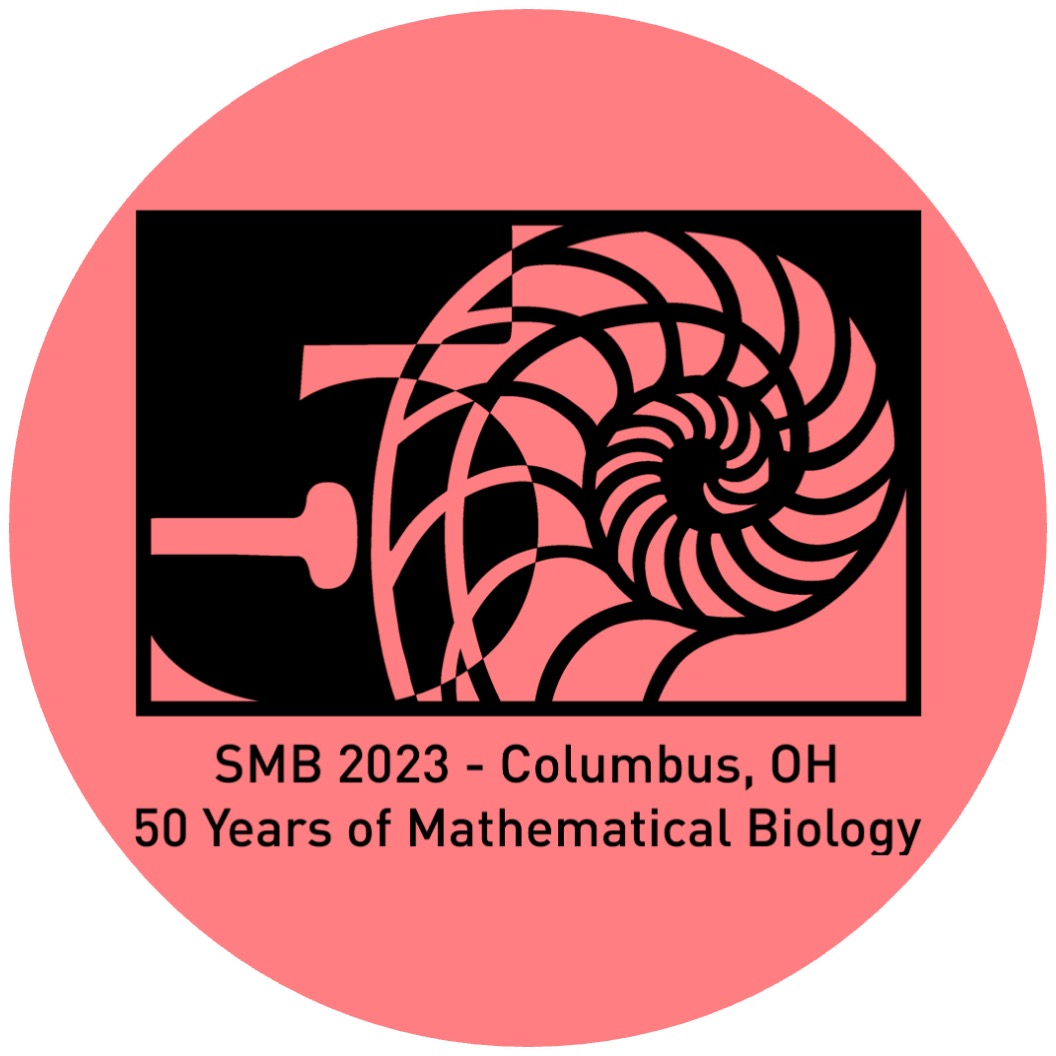
Share this
Jason E. Shoemaker
University of Pittsburgh"Sex-specific immunoregulation: Computational modeling approaches to determine why biological females may experience greater inflammation during influenza infection"
Elizabeth R Duke
Fred Hutchinson Cancer Center"Intrahost mathematical modeling of CAR T cells for HIV cure"
Vitaly V. Ganusov
University of Tennessee"Using mathematical modeling to determine pathways of Mycobacterium tuberculosis dissemination in mice"
Alexis Hoerter
Purdue University"Agent Based Model Investigating Latent and Naïve In Vitro M. Tuberculosis Infection Dynamics"
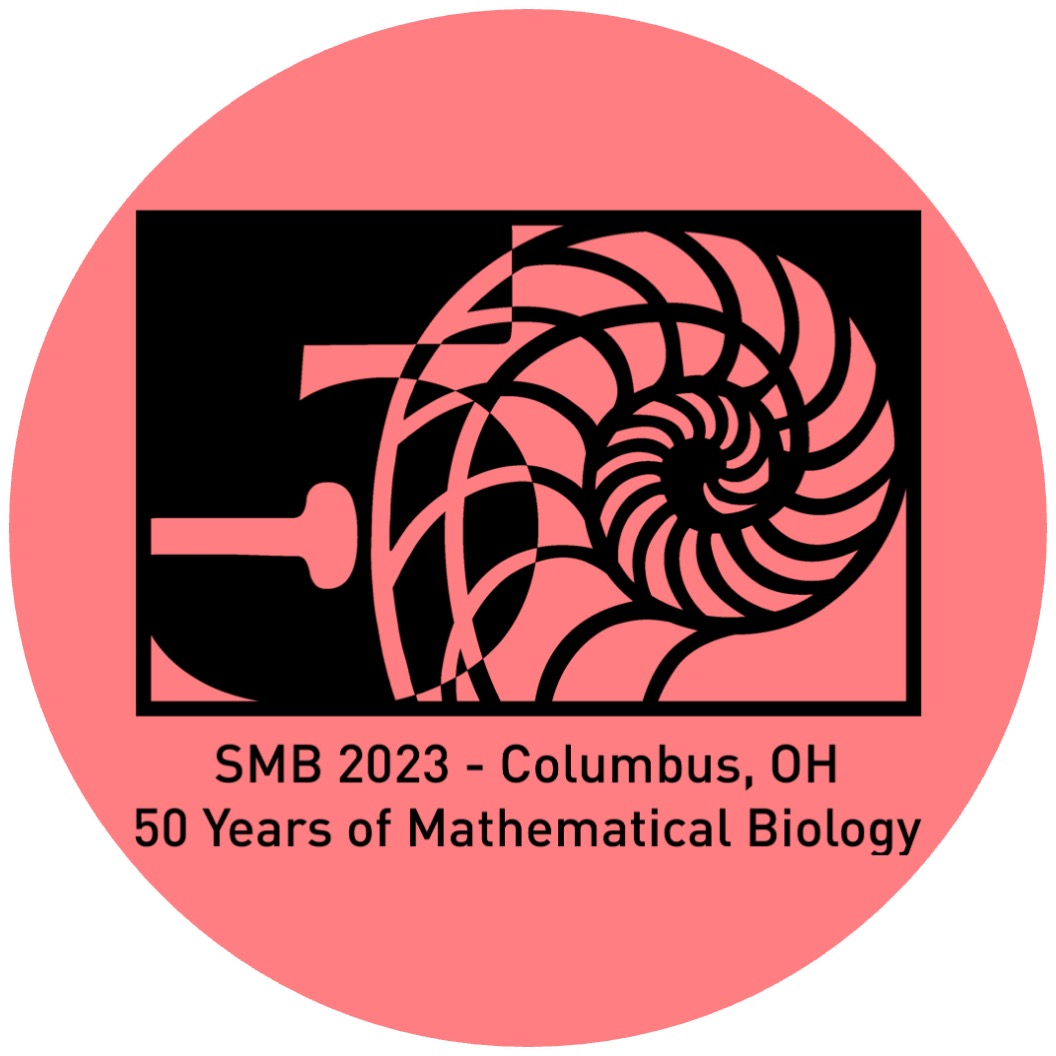