CT01 - ONCO-1 Ohio Staters Traditions Room (#2120) in The Ohio Union
ONCO Subgroup Contributed Talks
Monday, July 17 at 2:30pm
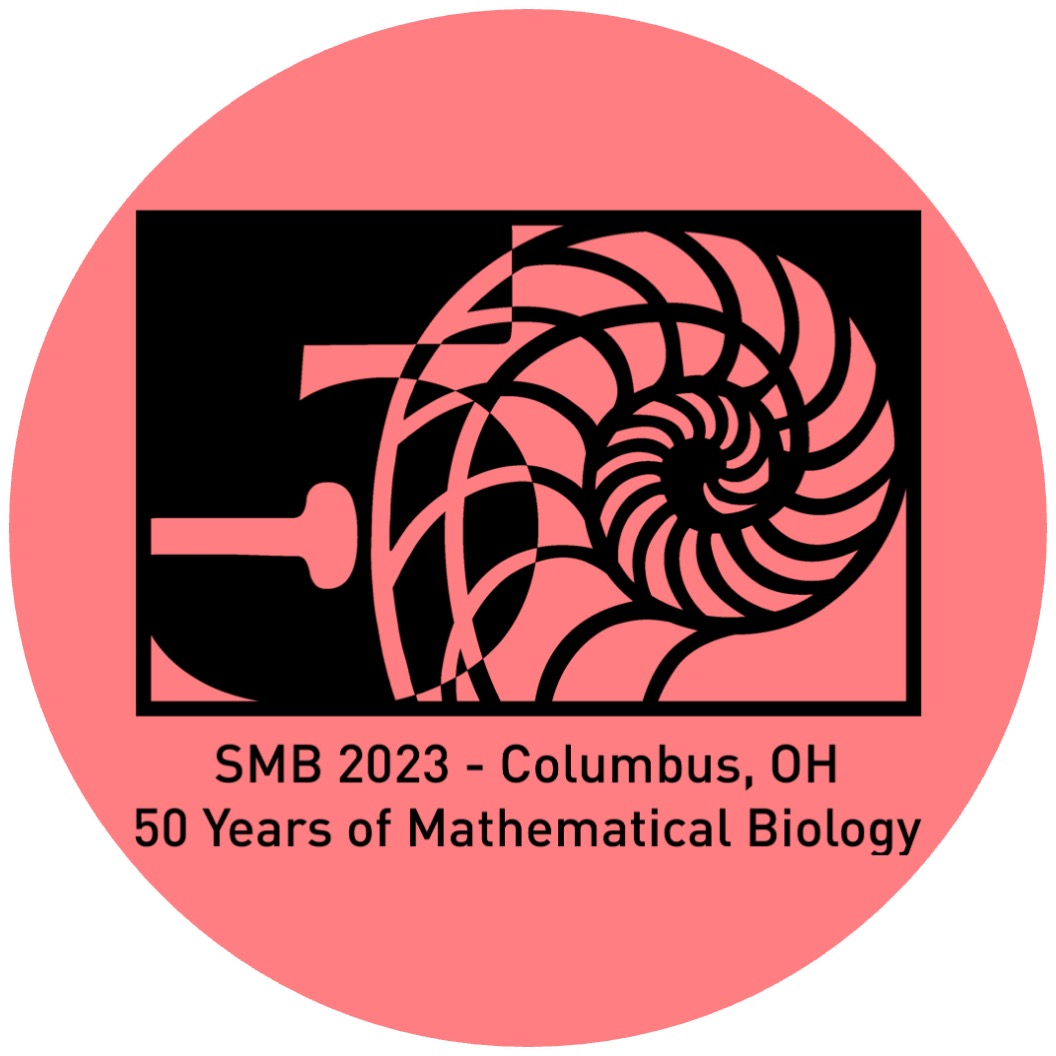
Share this
Daniel Glazar
Moffitt Cancer Center & Research Institute"A simulation-based sample size analysis of a joint model of longitudinal and survival data for patients with glioma"
Guillermo Lorenzo
University of Pavia, Italy"Personalized MRI-informed predictions of prostate cancer growth during active surveillance"
Jason T. George
Texas A&M University"Physical Modeling of the T Cell-Peptide Interaction: Toward Predictive T Cell Immunotherapy"
Jeffrey West
Moffitt Cancer Center"Markov models predict minimal residual disease in Adult B-Lymphoblastic Leukemia"
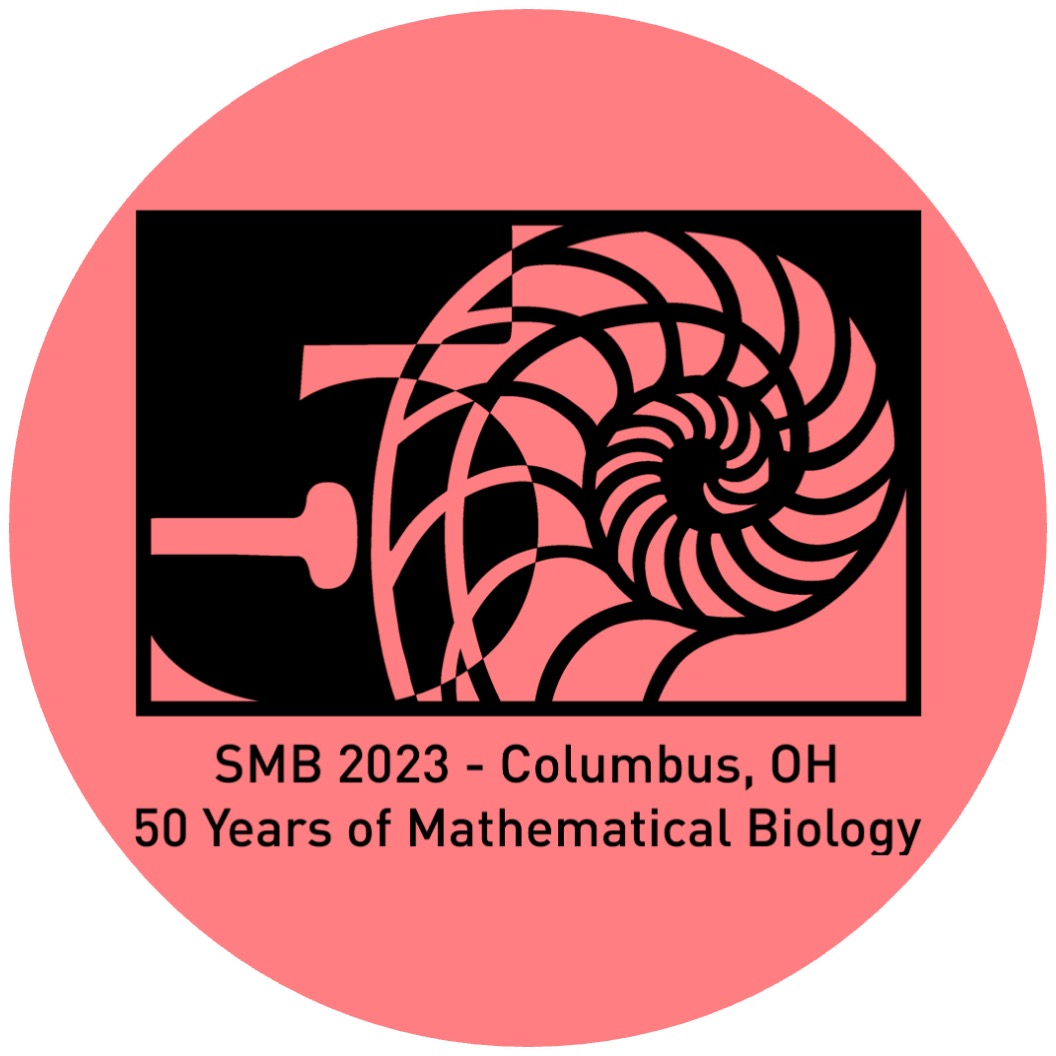